VectorDB tool
VectorDB is a robust tool enabling you to generate embeddings for your content, such as websites or extended texts, and store them in your dedicated VectorDB. Once stored, SWE facilitates the retrieval of data from these embeddings, allowing your application's model to utilize it for additional context. Learn more about the tool concept here
Pay attention
Please note that to achieve this successfully, you must use the same embedding model in SWE that you used to store the data.
Supported VectorDB's
Currently, SWE offers support exclusively for pgvector (PostgreSQL), but keep an eye out—we're planning to bring in support for more varieties very soon!
Using the UI
This guide will walk you through creating a VectorDB tool using the user interface (UI) client. VectorDB tools help connect your system to a database containing vector embeddings, which can be used to enrich prompts and improve model understanding.
- Add a New Tool:
- Click the "+ Tool" button. This opens a menu where you can choose the type of tool you want to add.
- Select "VectorDB tool" to begin setting up the connection.
- Name Your Tool: Assign a descriptive name to your tool. This helps the model understand the context in which the data will be used. For example, "Product Embeddings" or "Customer Search Vectors" would be good choices.
- Choose the VectorDB Type: Currently, only "pgvector" is supported. This refers to the specific type of database technology used for your VectorDB. If you're unsure, consult your system administrator.
- Connect to Your Database: Enter the connection string. This string contains the information needed to connect to your VectorDB instance. You can typically find this string in your database configuration details.
Provide the embedding table name. This specifies the table within your VectorDB that stores the actual vector embeddings. - Describe Your tool: Consider adding a comprehensive description of your tool. This helps the model understand the purpose and context of your database. Explain what kind of data the embeddings represent (e.g., product descriptions, user profiles) and how they are used in your system. This information can improve the relevance and accuracy of the prompts generated by the model.
- Link your embedding model : Provide information about the specific model here. Please pay attention that to achieve this successfully, you must use the same embedding model in SWE that you used to store the data.
By following these steps, you'll successfully set up a VectorDB tool within the UI. This will allow your system to leverage the power of vector embeddings, potentially leading to improved performance and more insightful results.
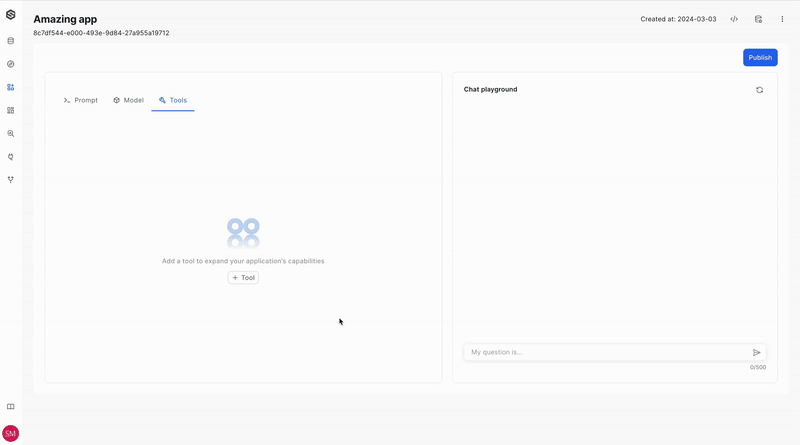
Using the SDK
To begin crafting your VectorDB tool with the help of the SDK, you'll find the provided code snippets below as an invaluable guide. These snippets will lead you through the necessary steps to integrating the VectorDB tool into your workflow
Step 1: Create embedding model
OpenAI embedding model
from superwise_api.models import OpenAIEmbeddingModel, EmbeddingModelProvider, OpenAIEmbeddingModelVersion
embedding_model=OpenAIEmbeddingModel(
provider=EmbeddingModelProvider.OPEN_AI,
version=OpenAIEmbeddingModelVersion.TEXT_EMBEDDING_ADA_002,
api_key="Your API key"
)
Google Vertex AI Model Garden embedding model
from superwise_api.models import EmbeddingModelProvider, VertexAIModelGardenEmbeddingModel
embedding_model=VertexAIModelGardenEmbeddingModel(
provider=EmbeddingModelProvider.VERTEX_AI_MODEL_GARDEN,
project_id="Your project id",
endpoint_id="Your endpint id",
location="us-central1",
service_account={SERVICE_ACCOUNT}}
)
Step 2: Create tool
Tool creation includes the following details:
- Assign a meaningful name to your tool. The chosen name will aid the model in recognizing the context for which the data is intended.
- Connect to Your Database: Enter the connection string. This string contains the information needed to connect to your VectorDB instance. You can typically find this string in your database configuration details.
Provide the embedding table name. This specifies the table within your VectorDB that stores the actual vector embeddings. - Provide a comprehensive description of the tool. Elaborate on the database’s purpose and its operational context. This description helps the model to contextualize the data, thereby enhancing the relevance and accuracy of the system-generated prompts
- Link your embedding model : Provide information about the specific model here. Please pay attention that to achieve this successfully, you must use the same embedding model in SWE that you used to store the data.
from superwise_api.models import ToolDef, ToolType, ApplicationConfigPayload, \
ToolConfigPGVector, EmbeddingModelProvider, \
VertexAIModelGardenEmbeddingModel
vectordb_tool =ToolDef(
name="Tool name",
description="Describe this tool for the LLM",
config=ToolConfigPGVector(
type=ToolType.PG_VECTOR,
connection_string="CONNECTION_STRING",
table_name="Your table name",
embedding_model=embedding_model
)
)
update_app_tools_payload = ApplicationConfigPayload(model=model, prompt=None,
tools=[vectordb_tool],
name="My application name")
updated_app = sw.application.put(str(app.id), update_app_tools_payload)
Test connection
SWE offers you the option to check the connection to your resources in any given time by using the following API call
Test connection to pgvector
POST app.superwise.ai/v1/applications/test-tool-connection
{
"type": "PGVector",
"connection_string": "",
"table_name": "",
"embedding_model": {
"provider": "VertexAIModelGarden",
"project_id": "",
"location": "",
"endpoint_id": "",
"service_account": {
"type": "service_account",
"project_id": "",
"private_key_id": "",
"private_key":"",
"client_email": "",
"client_id": "",
"auth_uri": "",
"token_uri": "",
"auth_provider_x509_cert_url": "",
"client_x509_cert_url":"",
"universe_domain":""
}
}
}
Updated about 1 year ago